Bias vs. Random Error: Hitting the Bullseye in Data Analysis

In the world of data analysis, accuracy is paramount. Whether you’re a data scientist, researcher, or business analyst, understanding the difference between bias and random error is crucial for drawing reliable conclusions. These two concepts often confuse beginners but mastering them can significantly improve your data analysis skills. Let’s dive into what they mean, how they differ, and how to minimize their impact on your results.
What is Bias in Data Analysis?
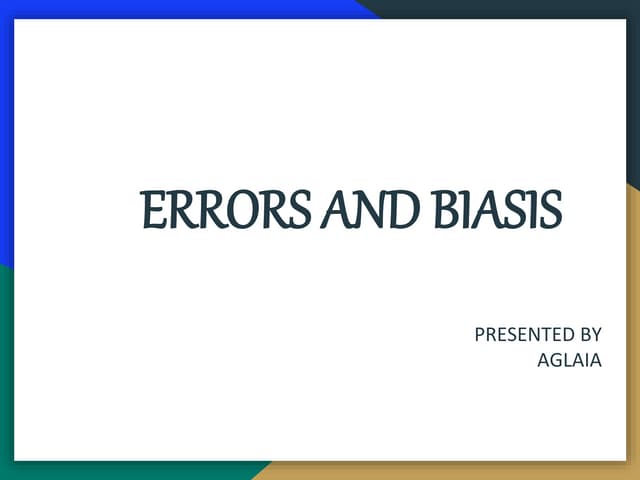
Bias refers to systematic errors that consistently skew results in one direction. Unlike random errors, bias doesn’t average out over time. It arises from flawed data collection methods, poor sampling techniques, or inherent limitations in measurement tools. For instance, if a survey only targets a specific demographic, the results will be biased toward that group, failing to represent the broader population.
📊 Note: Bias is harder to detect because it appears consistent and logical, often masquerading as accurate data.
What is Random Error?
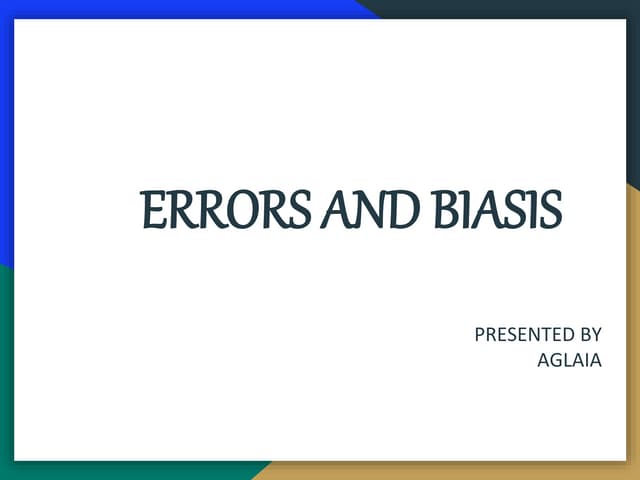
Random error, on the other hand, is unpredictable and unpredictable. It occurs due to natural variability in measurements or data collection processes. For example, if you measure the height of a person multiple times, slight differences in readings are random errors. Unlike bias, random errors tend to cancel each other out when you increase the sample size.
Aspect | Bias | Random Error |
---|---|---|
Nature | Systematic | Unpredictable |
Impact | Consistent skewing | Cancels out over time |
Cause | Flawed methods | Natural variability |

How to Identify and Minimize Bias

Identifying bias requires a critical examination of your data collection and analysis methods. Here’s a checklist to help:
- Review Sampling Techniques: Ensure your sample is representative of the population.
- Check Measurement Tools: Verify that tools are calibrated and reliable.
- Analyze Data Sources: Avoid relying on single or biased sources.
- Question Assumptions: Challenge underlying assumptions in your analysis.
Strategies to Reduce Random Error

Minimizing random error involves increasing precision and sample size. Consider the following steps:
- Increase Sample Size: Larger samples reduce the impact of random fluctuations.
- Improve Measurement Techniques: Use high-quality tools and standardized procedures.
- Repeat Measurements: Multiple readings can help identify and average out errors.
Bias vs. Random Error: Key Takeaways

Understanding the difference between bias and random error is essential for accurate data analysis. While bias systematically skews results, random error introduces variability that can be mitigated with larger samples. By identifying and addressing both, you can ensure your findings are reliable and actionable.
What is the main difference between bias and random error?
+Bias is a systematic error that consistently skews results, while random error is unpredictable and tends to cancel out over time.
How can I minimize bias in my data analysis?
+Review sampling techniques, check measurement tools, analyze data sources, and question underlying assumptions.
Why is random error easier to manage than bias?
+Random error can be reduced by increasing sample size and improving measurement techniques, whereas bias requires addressing flaws in methodology.
By focusing on these principles, you’ll be better equipped to hit the bullseye in your data analysis efforts, ensuring your insights are both accurate and trustworthy. (data accuracy,error reduction,statistical analysis)