Distribution-Free Causal Inference: Unlocking Reliable Insights

<!DOCTYPE html>
In today’s data-driven world, understanding the causal relationships between variables is crucial for making informed decisions. However, traditional causal inference methods often rely on strict assumptions about data distributions, which can limit their applicability. Enter distribution-free causal inference, a groundbreaking approach that removes these constraints, enabling more robust and reliable insights. Whether you’re a data scientist, researcher, or business leader, this method can revolutionize how you analyze and interpret data. (causal inference, data analysis, decision-making)
What is Distribution-Free Causal Inference?
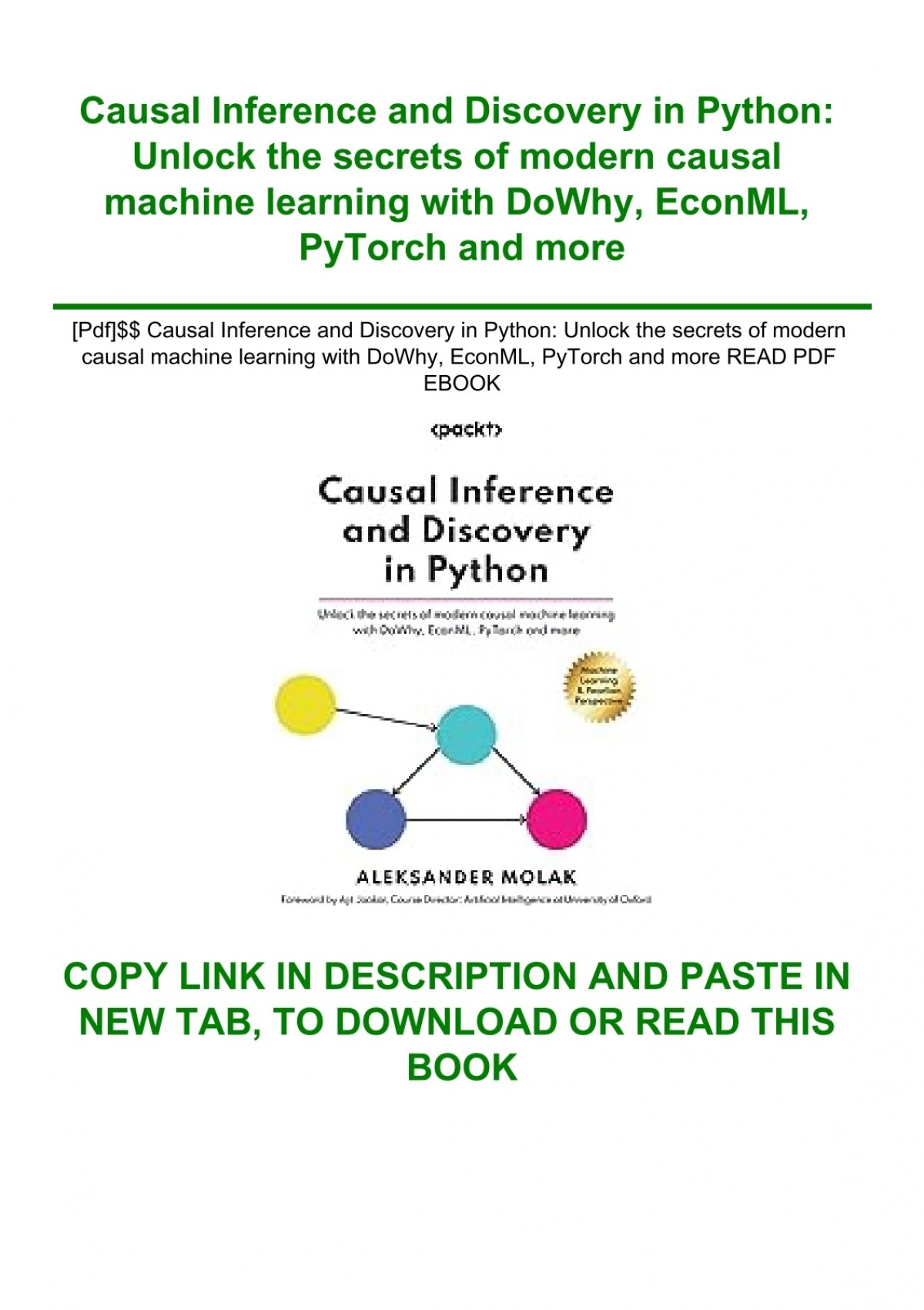
Distribution-free causal inference is a statistical method that identifies causal relationships without assuming a specific data distribution. Unlike traditional approaches, it doesn’t rely on parametric models or normality assumptions, making it versatile across various datasets. This flexibility ensures that insights remain valid even when data patterns are unpredictable or complex. (statistical methods, parametric models, data patterns)
Why is Distribution-Free Causal Inference Important?

The importance of this method lies in its ability to handle real-world data challenges. Traditional causal inference often fails when data violates assumptions, leading to biased or unreliable results. Distribution-free methods, however, provide a robust alternative, ensuring accurate insights even in noisy or non-standard datasets. This makes it invaluable for industries like healthcare, finance, and marketing, where data complexity is the norm. (real-world data, biased results, healthcare analytics)
Key Benefits of Distribution-Free Causal Inference
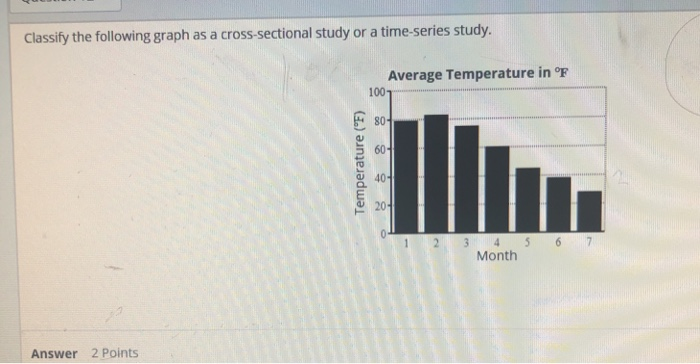
- Robustness: Works effectively with noisy or non-standard data.
- Flexibility: Applies to various datasets without distribution assumptions.
- Accuracy: Reduces bias and improves reliability of causal insights.
- Scalability: Suitable for large-scale and complex data environments.
(robustness, flexibility, accuracy, scalability)
How to Implement Distribution-Free Causal Inference
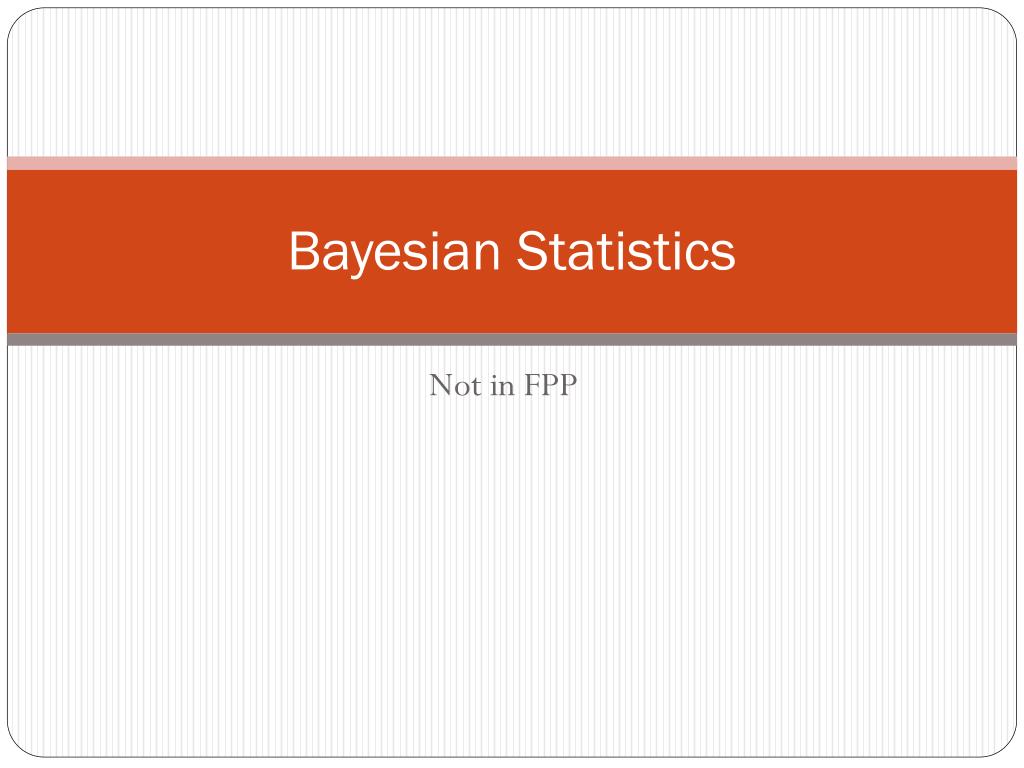
Implementing this method involves several steps:
- Data Collection: Gather relevant data while ensuring it’s representative of the population.
- Model Selection: Choose a distribution-free causal inference model, such as those based on rank statistics or kernel methods.
- Analysis: Apply the model to identify causal relationships without assuming data distributions.
- Validation: Test the model’s robustness using cross-validation or bootstrapping techniques.
📌 Note: Always ensure your data is clean and preprocessed before applying any causal inference method.
(data collection, model selection, causal relationships, model validation)
Applications of Distribution-Free Causal Inference
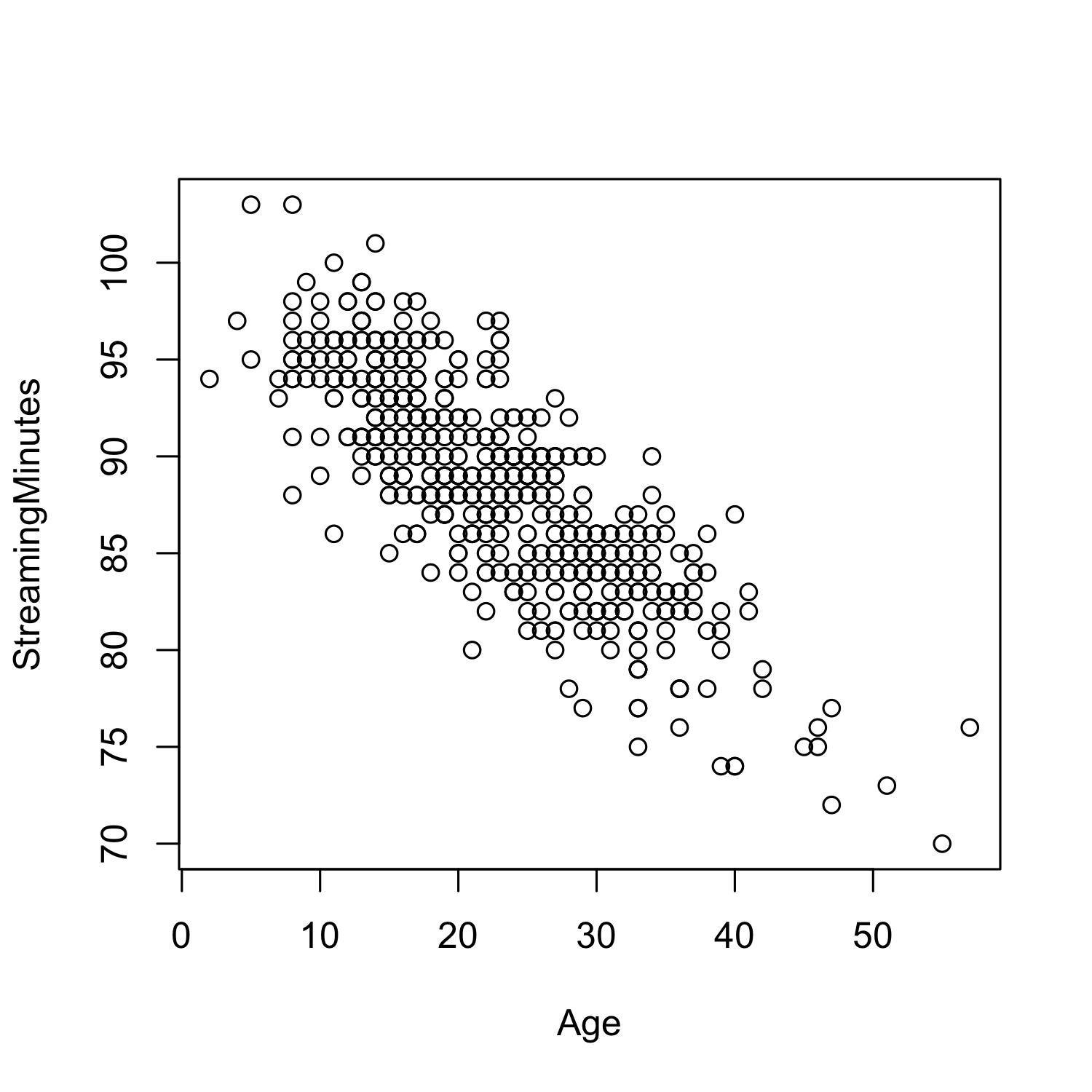
This method has wide-ranging applications across industries:
Industry | Application |
---|---|
Healthcare | Identifying treatment effects in clinical trials |
Finance | Assessing the impact of policy changes on market trends |
Marketing | Measuring the effectiveness of ad campaigns |

(healthcare analytics, financial analysis, marketing effectiveness)
Checklist for Implementing Distribution-Free Causal Inference
- Ensure data is representative and clean.
- Select an appropriate distribution-free model.
- Validate results using robust techniques.
- Interpret findings in the context of your business goals.
(data cleaning, model selection, result validation, business goals)
Distribution-free causal inference is a powerful tool for unlocking reliable insights from complex data. By removing the constraints of traditional methods, it offers a flexible and robust approach to causal analysis. Whether you’re tackling healthcare challenges, financial predictions, or marketing strategies, this method can help you make data-driven decisions with confidence. Embrace distribution-free causal inference and transform the way you analyze data today. (data-driven decisions, causal analysis, healthcare challenges)
What makes distribution-free causal inference different from traditional methods?
+It doesn’t rely on specific data distribution assumptions, making it more flexible and robust.
Can distribution-free causal inference handle large datasets?
+Yes, it’s scalable and suitable for complex, large-scale data environments.
What industries benefit most from this method?
+Healthcare, finance, and marketing are among the top industries benefiting from its robustness and accuracy.