Reinforcement Learning Optimizes Brain Drug Delivery Strategies

Reinforcement Learning (RL) is revolutionizing the way we approach brain drug delivery strategies, offering precise and optimized solutions for complex neurological conditions. By leveraging AI algorithms, RL enables the development of targeted therapies that maximize efficacy while minimizing side effects. This innovative approach is particularly crucial in treating brain disorders, where traditional methods often fall short due to the blood-brain barrier and the brain's intricate anatomy. In this post, we'll explore how RL is transforming drug delivery, its applications, and the future potential of this technology. (brain drug delivery, reinforcement learning in neuroscience, AI in medicine)
Understanding Reinforcement Learning in Drug Delivery
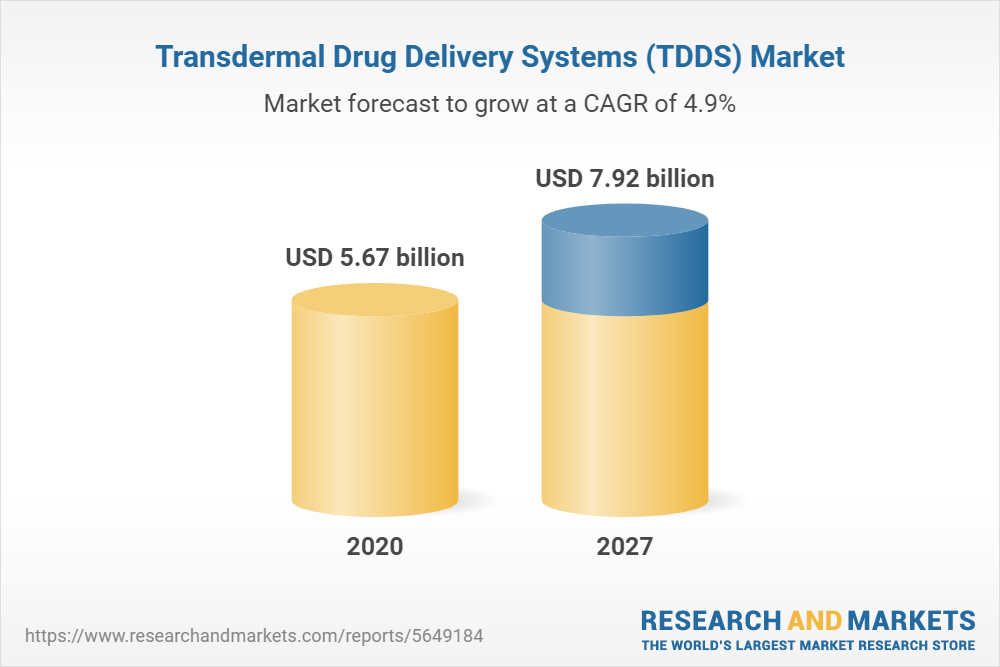
Reinforcement Learning is a subset of machine learning where algorithms learn to make decisions by performing actions and receiving feedback in the form of rewards or penalties. In the context of brain drug delivery, RL models simulate various delivery methods, such as nanoparticles or convection-enhanced delivery, to identify the most effective approach.
- Key Components of RL in Drug Delivery:
- Agent: The decision-maker (e.g., drug delivery system)
- Environment: The brain’s physiological conditions
- Reward: Optimal drug concentration at the target site
By iteratively refining strategies, RL ensures that drugs reach their intended targets with unparalleled precision. (reinforcement learning algorithms, drug delivery systems, AI in healthcare)
Applications of RL in Brain Drug Delivery
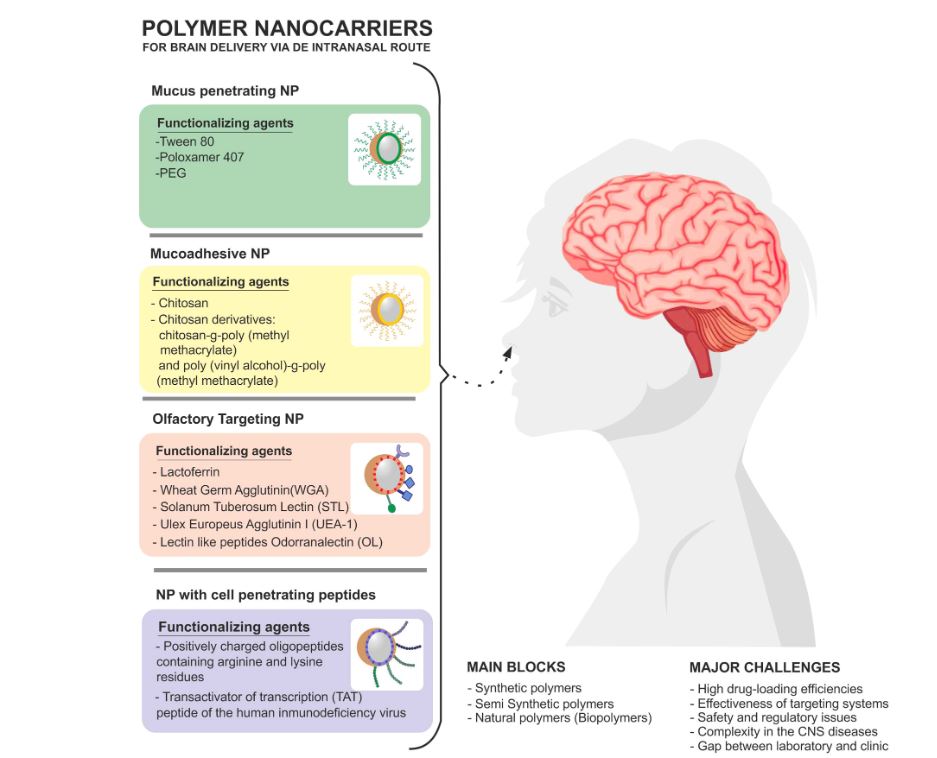
Treating Neurodegenerative Diseases
RL is particularly promising for conditions like Alzheimer’s and Parkinson’s, where targeted drug delivery is essential. By optimizing nanoparticle design and release mechanisms, RL enhances drug penetration across the blood-brain barrier.
Personalized Medicine
RL enables the customization of drug delivery strategies based on individual patient data, such as brain anatomy and disease progression. This personalized approach improves treatment outcomes and reduces adverse effects.
Application | Benefit |
---|---|
Alzheimer’s Treatment | Improved drug penetration |
Personalized Therapy | Tailored treatment plans |
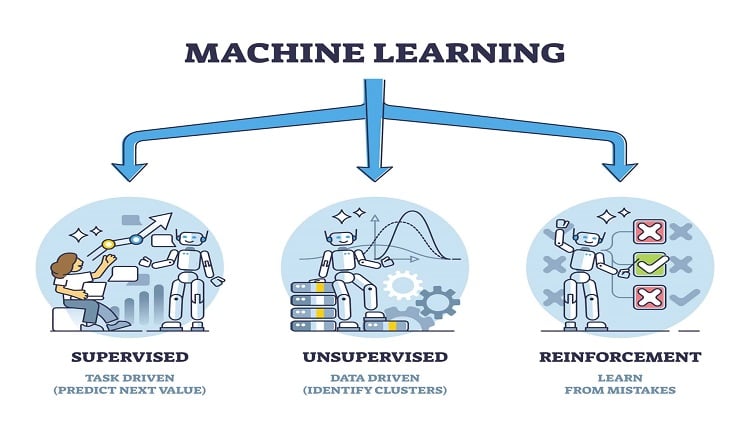
These advancements highlight RL’s potential to address long-standing challenges in neuroscience. (neurodegenerative diseases, personalized medicine, AI in neuroscience)
Challenges and Future Prospects

While RL shows immense promise, challenges such as data scarcity and computational complexity remain. However, ongoing research and advancements in AI are paving the way for more efficient and scalable solutions.
💡 Note: Collaborations between AI researchers and neuroscientists are critical to overcoming these hurdles and translating RL into clinical practice.
The future of RL in brain drug delivery looks bright, with potential applications in real-time monitoring and adaptive therapy. (challenges in AI, future of drug delivery, neuroscience research)
Summary and Checklist
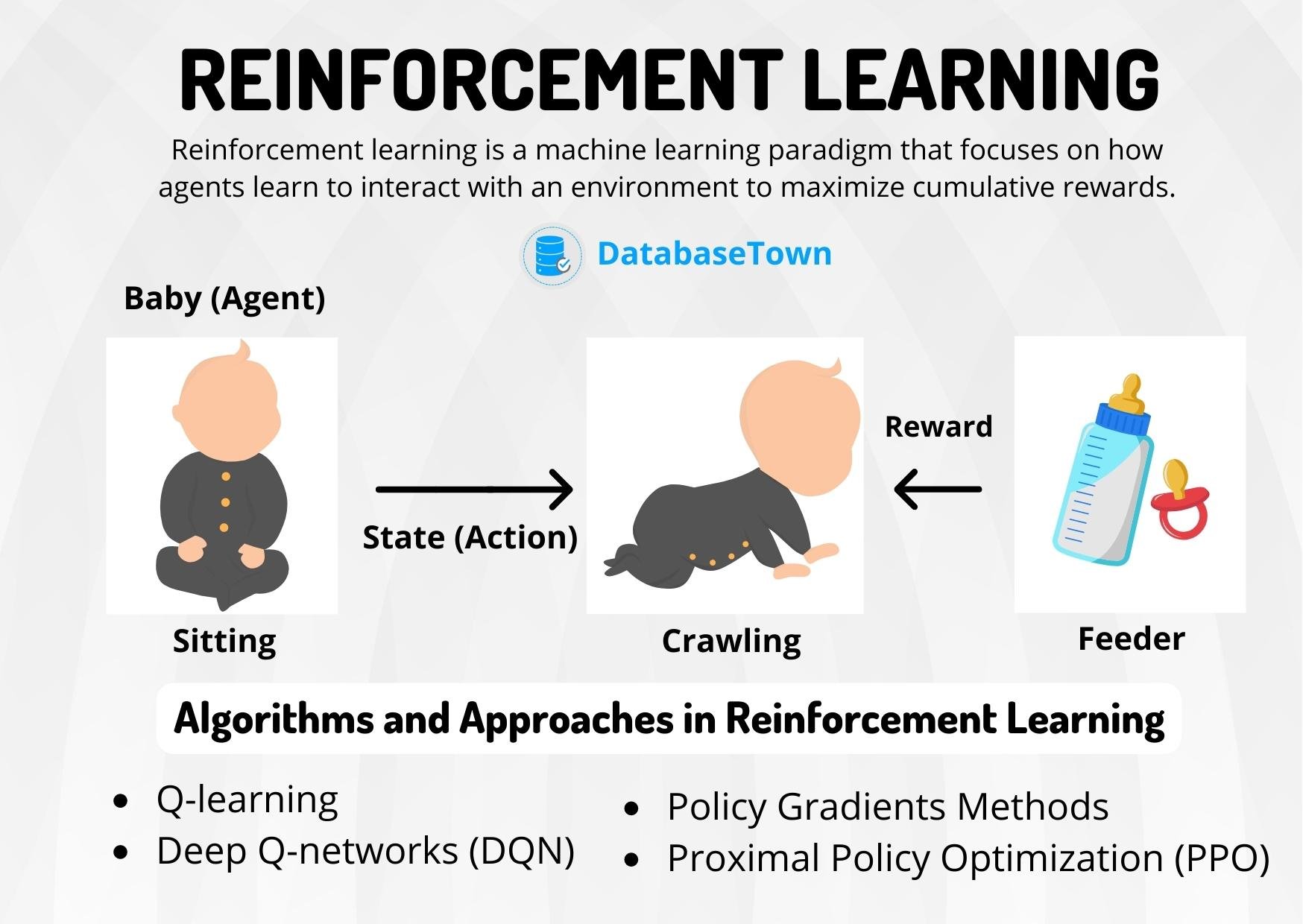
Reinforcement Learning is optimizing brain drug delivery strategies by providing precise, personalized, and efficient solutions. Here’s a quick checklist to recap:
- Understand RL’s role in simulating and optimizing drug delivery methods.
- Explore applications in treating neurodegenerative diseases and personalized medicine.
- Acknowledge challenges and the importance of interdisciplinary collaboration.
By embracing RL, we can unlock new possibilities in neuroscience and improve patient outcomes. (brain drug delivery strategies, AI innovations, healthcare technology)
What is Reinforcement Learning in drug delivery?
+Reinforcement Learning is an AI technique where algorithms optimize drug delivery strategies by learning from feedback, ensuring precise and effective treatment.
How does RL improve brain drug delivery?
+RL enhances drug delivery by simulating and refining methods to overcome barriers like the blood-brain barrier, ensuring targeted and efficient treatment.
What are the challenges of using RL in neuroscience?
+Challenges include data scarcity, computational complexity, and the need for interdisciplinary collaboration to translate RL into clinical practice.
Reinforcement Learning is at the forefront of transforming brain drug delivery strategies, offering hope for patients with complex neurological conditions. By addressing current challenges and fostering collaboration, we can harness RL’s full potential to revolutionize healthcare. (reinforcement learning, brain drug delivery, AI in neuroscience)